Aha, I get it now. You’re talking about systematic underpredictions of winrates in FIDE rated games between lower rated and higher rated players, which Sonas pointed out in 2002:
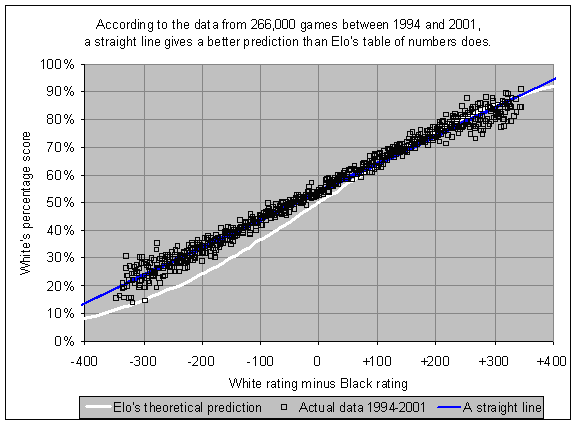
In 2011 he points out that this can be fixed by adjusting the Elo formula a bit. Instead of defining a 400 rating gap as corresponding to 1:10 odds, it could define a 480 rating gap as corresponding to 1:10 odds, and then the predictions would fit the observations:
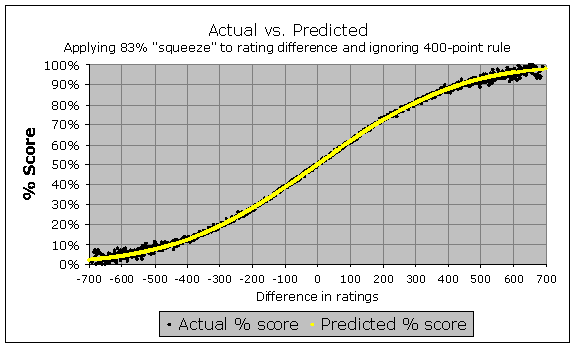
While this may “work” in the case of these FIDE rated games, it does feel like a hack to me. It’s like ad hoc adjusting all weather forecasts 2 degrees downward to make it fit observations better.
Instead of applying such a hack, I think it would be better to investigate what might be causing the discrepancy. Perhaps adjusting some other parameters in the system would also fix the issue, while having a better theoretical basis than the hack.
For instance, the observations show that lower rated players tend to win more often against higher rated players than predicted from the rating difference. This could be caused by a tendency of lower rated players to be systematically underrated. And that could be caused by ratings of upcoming lower rated players catching up too slowly to track their actual improvement. And that could be caused by the K factor of these games being too small and/or by a lack of sufficient rating point injections into the system to prevent deflation from improving players.
I don’t know if those hypotheses are actually true for FIDE ratings, but I think it’s worth it to investigate those and potentially counter root causes for the issue, rather than applying the proposed hack.
Now, I don’t think EGF ratings have such a systematic discrepancy, at least not anymore since the 2021 retroactive update of the EGF rating system. (EGF ratings are not Elo ratings, but under the hood the EGF rating system actually does use the Elo algorithm on implied Elo ratings).
I was heavily involved in preparing that update and it was apparent to me that the original conversion had a prediction mismatch similar (much worse even) to what Sonas observed in FIDE ratings [1].
So I specifically aimed at shaping the Elo to rank conversion such that the winning predictions matched winning observations as closely as possible with a rather simple formula, see the β function at https://www.europeangodatabase.eu/EGD/EGF_rating_system.php#System. Together with some other parameter adjustments (such as the K factor and anti-deflationary rating point injections into the system), this resulted in a much better match between predicted and observed winrates [2].
As far as I understand, OGS did a similar thing (although perhaps not as elaborate) in its most recent major rating system update a couple of years ago.
[1] (before the update)
Note that the ratings on the horizontal axis are EGF ratings, not Elo ratings
[2] (after the update)
Note that the ratings on the horizontal axis are EGF ratings, not Elo ratings